The 10th international conference on Machine Learning and Artificial Intelligence applications,
taking place in person in Prague and online.
Machine Learning Prague 2025
– , 2025
Registration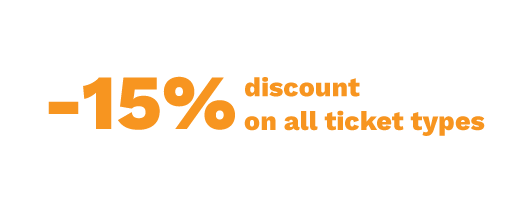
World class expertise and practical content packed in 3 days!
You can look forward to an excellent lineup of 45 international experts in ML and AI business and academic applications at ML Prague 2025. They will present advanced practical talks, hands-on workshops, and other forms of interactive content to you.
What to expect
- 1000+ Attendees
- 3 Days
- 45 Speakers
- 10 Workshops
Phenomenal Confirmed speakers
Practical & Inspiring Program
Friday
Workshops
O2 Universum, Českomoravská 2345/17a, 190 00, Praha (workshops won't be streamed)
Registration –
Room D2 | Room D3 | Room D4 | Room D6 | Room D7 | |
---|---|---|---|---|---|
–
coffee break – |
Utilizing Large Language Models for improved anti-tracking in web browsersRoom D2
Humera Noor Minhas, Digital Munich Online tracking remains a significant privacy concern for internet users. Current solutions while effective have limitations in terms of coverage maintenance and precision. This workshop aims to leverage the power of LLMs to create a more robust adaptive and efficient anti-tracking system. We will explore the architecture of an LLM-based anti-tracking system developing the data pipeline and exploring how these models can be fine-tuned to analyze network requests page content and user interactions in real-time. The system's ability to understand the semantic context of web elements allows for more accurate identification of tracking attempts reducing false positives while improving detection rates of sophisticated trackers. A key focus will be on the practical challenges of implementing such a system within the constraints of a web browser environment. We'll discuss strategies for optimizing LLM inference to meet the real-time demands of browsing balancing accuracy with performance. |
Accelerating AI Through Human Knowledge: Teaching to Imitate Experts and Win on the Race TrackRoom D3
Alexander Buchelt, St. Pölten University of Applied Sciences In this 3-hour hands-on workshop participants will explore the exciting world of Imitation Learning a powerful technique in artificial intelligence that allows agents to mimic expert behavior and excel in complex environments. Building on the fundamentals of Reinforcement Learning this workshop introduces the theory behind Imitation Learning and demonstrates how it can be applied to solve real-world problems efficiently. By guiding AI through expert demonstration imitation learning accelerates training especially in environments where traditional reinforcement learning might be time-consuming or difficult. Imitation Learning is crucial for AI systems that need to learn from limited data or human expertise such as autonomous driving robotics and gaming. In contrast to trial-and-error methods in reinforcement learning imitation learning allows models to replicate the strategies of experienced individuals drastically reducing training time and improving performance. Attendees will gain a deep understanding of how this approach combines the best of both supervised and reinforcement learning creating smarter faster decision-making systems. |
Introduction to Algorithmic Trading: Hands-On Strategy Implementation with Real-World DataRoom D4
Szymon Bubak, Jiai Algorithmic trading has become a cornerstone of financial markets with automation and data-driven strategies driving the majority of transactions. This workshop provides a comprehensive hands-on introduction to the world of algorithmic trading aimed at students and professionals interested in financial markets who want to move beyond academic exercises and engage with real-world scenarios. Participants will develop a trading strategy using real-world data such as Bitcoin prices or stocks from the S&P 500 with the initial focus on achieving profitability under the assumption of no transaction fees. The 3-hour session will guide participants through the end-to-end process of designing implementing and backtesting a deep learning-based trading strategy. The workshop will be structured as follows: - Data Preparation: Participants will learn how to source and preprocess financial data preparing it for model input. - Feature Extraction: We will introduce simple features from the data to feed into a deep learning model. - Loss Function Design: The workshop will cover how to design a loss function tailored to trading strategies and objectives. - Model Training: Participants will implement and train a deep learning model using PyTorch. The workshop will focus on how to properly train models in low-data environments ensuring the model generalizes effectively by employing robust train/validation/test splits. Backtesting: We will backtest the model’s performance allowing participants to understand its strengths and limitations in various market conditions. Rather than dividing the session into theoretical and practical segments the entire workshop will seamlessly integrate theory and implementation. Participants will work on a live trading scenario continuously applying new knowledge as they progress through the workshop. By the end they will have created a complete training pipeline—from data preparation to model training and backtesting. A critical aspect of this workshop will be understanding the practical challenges of implementing algorithmic trading strategies in real-world markets. We will discuss the limitations of deep learning models when applied to financial data including how to mitigate overfitting in low-data environments. Additionally participants will explore the impact of transaction fees slippage and other market inefficiencies learning how these factors affect profitability. The workshop is designed for participants with a basic understanding of Python and machine learning but no prior experience with algorithmic trading is required. By the end of the session attendees will have gained practical skills in designing and implementing a trading strategy along with valuable insights into the intricacies of algorithmic trading in financial markets. Participants will be provided with all necessary code templates and data and they are expected to bring their own laptops to engage fully in the hands-on aspects of the workshop. This workshop offers a unique opportunity to bridge the gap between theoretical learning and real-world financial applications equipping participants with the tools and knowledge to pursue further exploration in algorithmic trading. |
InstructLab: plug your knowledge into a model easilyRoom D6
Štefan Bunčiak, Red Hat During this hands-on exercise you will learn what is InstructLab and how you can leverage it to easily extend Large Language Models with your data and run them on your infrastructure. The tool makes it easy to download run and chat with models locally on your laptop. InstructLab is a fully open-source project from Red Hat and the MIT-IBM Watson AI Lab that introduces Large-scale Alignment for chatBots (LAB). The paper behind it: https://arxiv.org/abs/2403.01081 The LAB method is driven by taxonomies which are largely created manually and with care. For a taxonomy you supply InstructLab then can generate synthetic data used to train a model. Everyone who has experience with LLMs can greatly benefit from this workshop. We will create our own knowledge documents use InstructLab to generate synthetic data out of them train a model from the data and chat with them. |
Beyond Real-World Limitations - Mastering Synthetic Data Generation for Enhanced ML PerformanceRoom D7
Tomáš Sikora, Emplifi As machine learning pushes into new frontiers the demand for diverse and representative datasets frequently outpaces the availability of real-world data. This workshop explores a spectrum of advanced techniques for synthetic data generation from traditional methods to cutting-edge AI agent-driven approaches. By embracing the principles of data-centric AI we'll start with traditional data generation techniques and progress to innovative AI agent-driven strategies. Throughout the workshop we'll demonstrate techniques that promise to overcome limitations in real-world datasets reshape the data landscape in ML and rigorously evaluate the quality of the generated data. Workshop Overview: - Setting the Stage: The Data Challenge in ML We'll begin with a brief historical context touching on pre-LLM approaches to synthetic data generation. This background will highlight the impact of recent advancements and set the stage for our deep dive into modern techniques. - Data-Centric AI: A Paradigm Shift We'll explore how the data-centric AI movement is reshaping our approach to machine learning. Participants will learn why focusing on data quality and targeted synthetic data generation can often yield better results than simply increasing dataset size or model complexity. -Evolution of Synthetic Data Generation with AI -LLM-Powered Data Creation: Leveraging large language models for synthetic data generation. -Multi-Agent Systems: Advancing to manually designed agent ecosystems for nuanced and accurate data production. -Automated Agent Workflows: Exploring the cutting edge with self-optimizing agent interactions for superior data quality. -Robust Evaluation in the Data-Centric Paradigm We'll emphasize rigorous evaluation techniques aligned with data-centric AI principles ensuring the effectiveness of synthetic data in real-world ML applications. This workshop is ideal for ML practitioners researchers and data scientists looking to overcome data quality and scarcity challenges. Participants should have a basic understanding of machine learning concepts and some experience with programming in Python. By the end of this workshop attendees will have a comprehensive understanding of how data-centric AI principles can be applied to synthetic data generation from LLM-based techniques to state-of-the-art agent-driven approaches. They'll be equipped with practical skills to implement these strategies potentially revolutionizing how they tackle data-related challenges in ML projects. |
– |
|
||||
–
coffee break – |
3D reconstruction from Images and their applicationRoom D2
Varun Burde, CIIRC, Czech Technical University This workshop will delve into recent advances in 3D computer vision and provide participants with practical hands-on experience in generating 3D reconstructions from image data. Attendees will explore cutting-edge techniques including neural radiance fields (NeRFs) Gaussian splatting multi-view stereo (MVS) and structure from motion (SfM) for surface reconstruction. The session will cover the fundamentals of 3D reconstruction focusing on how modern algorithms transform 2D images into detailed and accurate 3D models. Additionally participants will learn the essential steps of dataset creation and optimization for training advanced 3D reconstruction methods. A key feature of the workshop will involve capturing a set of images of objects and demonstrating how to systematically collect and organize data to ensure high-quality 3D model generation. Attendees will gain experience in building datasets tailored to different 3D reconstruction techniques such as NeRF and Gaussian splatting and optimizing them for improved accuracy and visual fidelity. This workshop is ideal for researchers engineers and enthusiasts seeking to understand the latest in 3D vision technologies with applications ranging from augmented reality and robotics to digital content creation. |
Parallel Genetic Algorithms in PythonRoom D3
Jakub Tomasz Gnyp, International Centre for Theory of Quantum Technologies In this workshop we delve into the construction and implementation of parallel genetic algorithms (PGAs) using Python. Genetic algorithms (GAs) and evolutionary algorithms in general are powerful tools for solving optimization problems and when parallelized they offer significant speedups and efficiency improvements. Participants who learn PGAs will also be able to apply them in reinforcement learning. The workshop will have a limited amount of mathematics - instead the focus will be on both the idea behind PGAs and practical coding skills. Starting with the PyGAD library its uses and limitations will be discussed and presented with easy-to-understand examples. Later key aspects of parallel programming will be introduced such as recognizing CPU- and I/O-bound operations and the use of processes and threads respectively. Global lock in Python will be addressed as well as racing conditions. Therefore a basic understanding and implementation of locks barriers flags and shared memory in general will be achieved. To illustrate the practical applications of parallel genetic algorithms apart from minor examples the workshop features three major case studies. The first involves solving a labyrinth demonstrating how a parallel genetic algorithm can efficiently navigate complex search spaces and de facto interact with an environment. Participants will observe how the parallelization of GAs can lead to faster convergence on optimal paths compared to sequential approaches. Diversity in population will be addressed as well. The second case study explores the application of parallel genetic algorithms in quantum cryptography. In this domain GAs can optimize parameters for quantum key distribution protocols enhancing especially efficiency. By parallelizing the algorithm we can tackle the computational challenges of the vast solution spaces inherent in quantum cryptographic systems. The BB84 protocol will be the protocol in question explained without the quantum mechanics' mathematical rigor and the essentials of the protocol will already be implemented. The third and last case study will be a neural network in which hyperparameters will be optimized by a PGA in a Genetically Reinforced Learning scheme. Knowing how the PGA may interact with an environment and work on even very complicated functions this optimization task will be an easy step for those who have already seen the neural network. By the end of the workshop participants will have a solid understanding of how to implement and apply parallel genetic algorithms in Python with practical insights into their strengths and limitations. They will be equipped with the knowledge to extend these techniques to other domains fostering innovation in computational problem-solving. |
Real-Time Anomaly Detection and Alerting in Financial Markets Using Stream ProcessingRoom D4
Tomáš Neubauer, Quix In the world of financial markets the ability to detect and act on anomalies in real-time is crucial. This workshop will explore how to build a stream processing system that not only detects rapid changes in stock prices but also calculates key stock market indicators like the Relative Strength Index (RSI) Moving Average Convergence Divergence (MACD) or Bollinger Bands in real-time. Attendees will learn how to calculate these indicators in real-time to identify potential buy or sell signals and trigger instant alerts such as Slack messages to notify users of significant market movements or even directly call API to buy/sell instruments. At the end we will discuss and later build a stream processing pipeline in the IDE using the ML model. |
A practical guide to LLM-based AI agentsRoom D6
Philipp Wendland, Deloitte Consulting This hands-on workshop is designed to provide participants with an in-depth practical understanding of how to leverage Large Language Models (LLMs) to create intelligent AI agents. As the rise of generative AI continues to transform industries it’s becoming increasingly important for both AI professionals and business leaders to understand the capabilities and implementation strategies for LLM-based systems. The Deloitte AI Institute focusses on brining AI expertise to clients across all industries ranging from innovation over strategy to capability building and scaling. Phillips’s strong technical background in physics / computer science enables him to bridge the gap between business and technology. 1: Introduction to LLM-based agents - Overview of the concept and architecture of AI agents - Introduction to popular frameworks to expedite agent development 2: Hands-on implementation - Guide participants through building a simple LLM-based AI agent using a given framework - Allow for customisation to demonstrate the flexibility and effectiveness of AI agents 3: Industry-Application - Outlook on newest developments of AI agents across various industries - Outlook on the potential of generative Ai and AI agents in particular across industries By the end of the workshop participants will have a solid understanding of the concepts behind LLM-based AI agents and hands-on experience in building these systems themselves. Led by an experienced facilitator from Deloitte’s AI Institute this workshop promises to provide valuable skills and knowledge that participants can leverage in their careers. This workshop is ideal for AI practitioners developers and researchers eager to explore the latest advancements in generative AI and apply LLM-driven automation in their respective fields. |
Synthetic Data Generation for Embedding Model Fine-TuningRoom D7
Ondřej Finke, O2/Dataclair Retrieving information from documents in non-English and domain-specific languages presents a challenge for many organizations. While general embedding models are powerful they often fall short when dealing with specialized terminology not encountered in their training data. This workshop offers a practical approach to addressing these issues: using a combination of real and synthetic data to build robust datasets for fine-tuning open embedding models. The workshop consists of two parts. First we provide an overview of embedding models fine-tuning techniques and methods for generating synthetic data tailored to these approaches. In the second part participants will engage in a hands-on session to generate synthetic data for fine-tuning their own models. |
Saturday,
Workshops
O2 Universum, Českomoravská 2345/17a, 190 00, Praha (and on-line)
Registration from 9:00
Welcome to ML Prague 2025
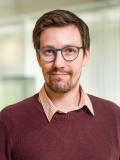
Evaluating LLM outputs with humans and LLMs
Ondřej Dušek, MFF Charles University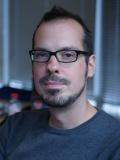
Data, your worst enemy?
Johan Loeckx, Vrije Universiteit Brussel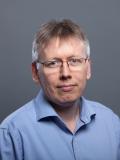
Lies, Damn Lies and Gen AI
Jon McLoone, Wolfram ResearchLUNCH & POSTER SESSION
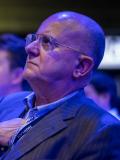
An introduction to protein structure prediction
Joseph Pareti, Joseph Pareti's AI consulting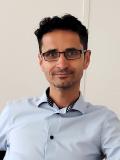
Mammography solved by AI?
Ivan Cimrák, University of Zilina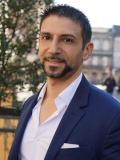
End-to-end Stroke imaging analysis, using reservoir computing-based effective connectivity, and interpretable AI
Alessandro Crimi, AGH University of KrakowCOFFEE BREAK
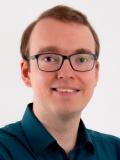
Attentive interpretable networks for scalable content recommendation in mobile games
Martin Dlask, King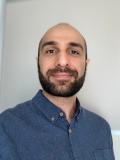
Evolution of Recommendation System: from ANN to Ensemble of Scorers
Raid Arfua, GR8 Tech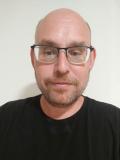
Estimating online behavior of ad hoc cohorts using context-dependent weighing of panel participants
Ariel Azia, SimilarwebCOFFEE BREAK
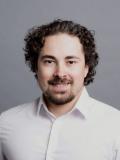
The Evolution of Virtual Buddy: From Concept to Deployment
Alexandr Vendl, Dataclair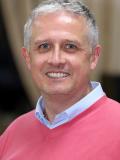
Intelligent Manufacturing Assistant Bot
Alexander Jesser, University HeilbronnPARTY
Sunday,
Conference day 1
O2 Universum, Českomoravská 2345/17a, 190 00, Praha (and on-line)
Doors open at 08:30
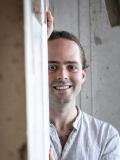
Solving adversarial attacks in computer vision
Stanislav Fort, Google DeepMind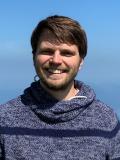
Training AI Models for Crime Scene Fingerprint Recognition
Jakub Sochor, Innovatrics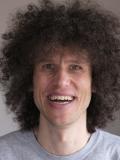
Understanding the neural networks through rule extraction
Tomas Pevny, Czech Technical UniversityCOFFEE BREAK
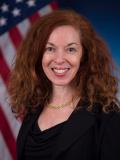
Distributed Collaborative AI
Hava Siegelmann, University of Massachusetts Amherst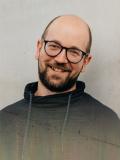
Advances and Challenges in Topic Modeling of Text Documents
Martin Neznal, Productboard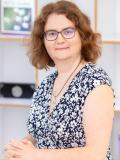
Towards Real-World Fact-Checking with Large Language Models
Iryna Gurevych, Technical University of DarmstadtLUNCH & POSTER SESSION
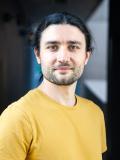
Towards Production-Ready Czech LLMs with Continuous Pretraining
Ondřej Filip, Seznam.cz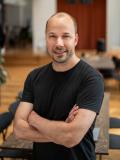
How to feed your LLMs with data from the web
Jan Čurn, Apify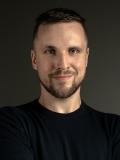
Fitting LLMs into a single GPU: Making neural networks smaller
Vladimir Macko, GrizzlyTech, former Google AICOFFEE BREAK
PANEL DISCUSSION
CLOSING REMARKS
Have a great time Prague, the city that never sleeps
You can feel centuries of history at every corner in this unique capital. We'll invite you to get a taste of our best pivo (that’s beer in Czech) and then bring you back to the present day at our networking event.
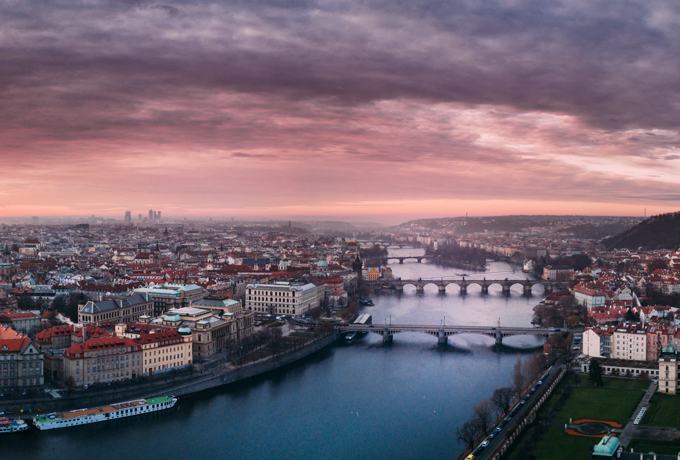
Venue ML Prague 2025 will run hybrid, in person and online!
The main conference as well as the workshops will be held at O2 Universum.
We will also livestream the talks for all those participants who prefer to attend the conference online. Our platform will allow interaction with speakers and other participants too. Workshops require intensive interaction and won't be streamed.
Conference building
O2 Universum
Českomoravská 2345/17a, 190 00, Praha 9
Workshops
O2 Universum
Českomoravská 2345/17a, 190 00, Praha 9
Now or never Registration
Early Bird
Sold Out
-
Conference days € 270
-
Only workshops € 200
-
Conference + workshops € 440
Standard
152 Registrations Left
Late
Until sold out
-
Conference days € 320
-
Only workshops € 260
-
Conference + workshops € 520
What You Get
- Practical and advanced level talks led by top experts.
- Networking and drinks with speakers and people from all around the world.
- Delicious food and snacks throughout the conference.
They’re among us We are in The ML Revolution age
Machines can learn. Incredibly fast. Faster than you. They are getting smarter and smarter every single day, changing the world we’re living in, our business and our life. The artificial intelligence revolution is here. Come, learn and make this threat your biggest advantage.
Our Attendees What they say about ML Prague
Thank you to Our Partners
Strategic Partners
Platinum Partners
Gold Partners
Communities and Further support
Would you like to present your brand to 1000+ Machine Learning enthusiasts? Send us an email at info@mlprague.com to find out how to become a ML Prague 2025 partner. Our basic partnership offer can be found here.
Happy to help Contact
If you have any questions about Machine Learning Prague, please e-mail us at
info@mlprague.com
Organizers
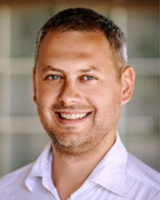
Jiří Materna
Scientific program & Co-Founder
jiri@mlprague.com
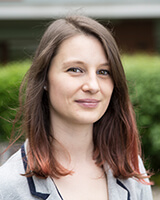
Teresa Pulda
Event production
teresa@mlprague.com
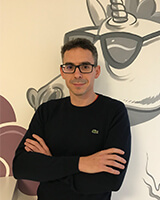
Gonzalo V. Fernández
Marketing and social media
gonzalo@mlprague.com
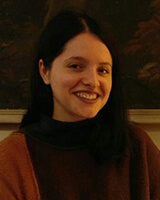
Jona Azizaj
Partnerships
jona@mlprague.com
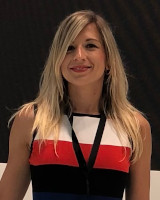
Ivana Javná
Speaker support
ivana@mlprague.com
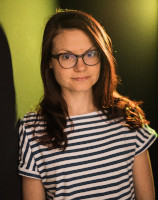
Barbora Toman Hanousková
Communication
barbora@mlprague.com
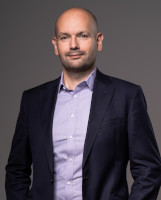
Jan Romportl
Moderator